The overall theme linking the following sections is one of multiple pests influencing a pathosystem. It is intended here that “multiple pests” may be interpreted with some flexibility to refer to ones that are taxonomically different (as in “The More the Merrier?”), functionally different (as in “Avirulence, Stress, and Fungal Sex”), or looser yet, different individuals of the same pest (as in the first example in “Spatiotemporal Interactions”). The final section—“We Need Information,
STAT(s)!” —presents one potential approach to probing plausibility for a biologically relevant factor not specified by a model that might be contributing certain variability, which may or may not be associated with multiple pests. Within each section, selected examples are presented to explore the biological relevance of multiple pests to epidemic development, whereas formulas are largely excluded.
As if out of a traditional storybook tale, our damsel in distress in a more cryptic situation is ‘Anastasia’, cultivar princess from the kingdom (read: species) of tomato. In the absence of third-party influences of physiological distraction, Anastasia is qualitatively resistant to
Tomato
spotted wilt virus (TSWV), a virus that has over 1,000 hosts (Sherwood et al. 2003). Enter into the equation
Tomato chlorosis virus (ToCV), a virus reported to have a natural host range of eight species (EPPO 2013) and to which Anastasia is normally susceptible (Garcia-Cano et al. 2006). When TSWV and ToCV are simultaneously introduced to Anastasia, its TSWV resistance remains. However, if Anastasia is first infected with ToCV around 10 days earlier, the resultant interference in plant defense responses acts as a predisposing gate opener to subsequently allow systemic TSWV movement (Garcia-Cano et al. 2006). In this case, resistance is overthrown by ToCV preinfection interfering with Anastasia’s characteristic defense mechanisms. Symptoms of ToCV infection alone can be irregular and commonly misidentified as abiotic or phytotoxic responses (EPPO 2005). In cases such as this, the relatively cryptic presence of an enabling infection can lead to dramatic synergistic system effects (i.e., here, systemic breakdown of TSWV resistance; see below in “Spatiotemporal Interactions” for additional related examples).
A question one can wonder is, how many times over the years have resistant varieties initially been considered individually defeated by a pathogen’s genetic counter punches when an accomplice to the crime may have been at large? In an interest to limit crop economic losses and enact corrective action as soon as possible, there may be a tendency for observed infection in previously resistant varieties to more likely be interpreted first as a breakdown in resistance in a single pathosystem interaction (e.g., pathogen-side mutation), rather than the potentially less suspected presence of third-party infection that may alone potentially exhibit ambiguous symptoms (assuming inoculum pressure, environmental conditions, etc. in a given observed case were not grossly advantaging infection). This may be more relevant on the first-responder/first-observer side of things, because formal resistance and resistance-breaking testing are common in current practice.
These two examples illustrate cases in which, generalizing, understanding and prediction of the development of a primary disease of interest can in cases greatly benefit from monitoring diseases or pests that might be less economically important themselves or less common items on the crop-management radar. The overall idea that disease intensity levels can be influenced by different pathogen genotypes goes back nearly a century with regard to cross-protection (Schumann and D’Arcy 2006), although in that case the situation is flipped in the sense that resistance can in some cases be gained from a separate previous infection. Similarly, the occurrence of disease as arising or being influenced by multiple pests or from a network of factors has been recognized in the literature for quite some time (Bergeson 1972; Fawcett 1931; Horsfall and Cowling 1979; Johnson 1990; Kranz and Jörg 1989; Savary and Zadoks 1992; Wallace 1978), and the overall principles behind integrated pest (or crop) management (i.e., multiple factors influencing disease) further reflect this with reports of some practices (e.g., rotation and time of planting) capable of being traced back hundreds to thousands of years (Orlob 1973; Zeigler et al. 1994).
Just as the presence of multiple components of a system can interact to alter the end product of disease severity, they can also influence steps along the way (parameters) within the disease cycle. In
Nicotiana benthamiana infected with both ToCV and
Tomato infectious chlorosis virus (TICV), Wintermantel et al. (2008) demonstrated how titers of TICV increased while titers of ToCV decreased. Conversely, levels of both viruses decreased in a different host,
Physalis wrightii (sharpleaf groundcherry) doubly infected by the same two viruses. Both ToCV and TICV are transmitted by whiteflies and can be found worldwide across all major areas of tomato production (EPPO 2013). The study further detailed how vector transmission efficiency of these viruses in individually compared with dually infected plants sometimes became substantially altered, additionally varying by vector and host species.
We can see from these examples how the amount of individual virus accumulation in a host, as well as the ability of the vector to successfully transmit these pathogens, can potentially be greatly influenced by the presence of specific pathogens or groups of pathogens in a manner that further depends on the host. The list of possible known types of interactions is much larger and includes modified infection rates (Tatineni et al. 2010) as well as fecundity and host preference of vectors (Srinivasan and Alvarez 2007). In situations such as these, we can readily imagine how forecasting models and decision support systems that perform very well based upon single-infection pathosystems could perform inadequately when applied to epidemics (unknowingly) exhibiting applicable co-infections. As the unevenness and variety of influential pathogens increases in a group of hosts in a shared area, the complexity of epidemic quantification (or its error when such factors are omitted) similarly may expand. Although the practical impact of varying system component levels under actual production conditions may be highly situational and not always associated with different economic outcomes, further work along these lines has the potential to be highly valuable given the costs associated with inadequate disease management in susceptible high-value crops.
Better understanding of the interplay of multiple pests (and other factors) in disease progression can be used to improve model development and regional applicability in areas where such situational synergistic agents (SSAs) are present. We may not need too much imagination to envision an age in which collecting data on SSAs becomes as commonplace as environmental monitoring to aid anticipation of threshold risk levels of targeted disease(s). Considering the disease triangle, the presence of SSAs can, after all, influence all three components (host, pathogen, and environment) as in
Figure 2. In this light, we can conceive of situations in which disease could be measured in terms of a disease triangle lattice (with each individual triangle-facet corresponding to a single pathosystem), although this would not necessarily simplify computational considerations and in some cases may only explain a relatively minor portion of additional disease variability.
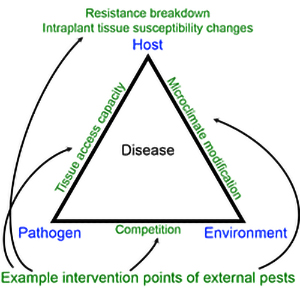 |
Figure 2. Disease triangle incorporating example points
in which pests external to a considered pathosystem, or
situational synergistic agents (SSAs), might influence
eventual disease levels. |
Avirulence, Stress, and Fungal Sex
In regard to plant disease management in agriculture, horticulture, forestry, and beyond, virulent or even pathogenic strains of pathogens are those that are implicitly of immediate concern when managing crops to reduce disease-related losses. If on a given variety a strain is avirulent, on the other hand, it does not cause disease and therefore is not the explicit target of further disease control measures. This may give the impression that avirulent strains have little relevance toward current or future disease losses. However, this too may present certain challenges (apart from considering virulence-gaining mutations of the same strain).
An example here demonstrating the relevant importance of avirulent strains is
Zymoseptoria tritici, causal agent of Septoria tritici blotch in wheat (McDonald et al. 2015). In nature,
Z. tritici produces both asexual conidia that are locally splash-dispersed and airborne sexual ascospores, the latter of which generally serve as primary inoculum for an epidemic in a given field (Ponomarenko et al. 2011). One of many fungi to do so (Thrall et al. 2016),
Z. tritici populations exhibit virulence heterogeneity, with some strains being virulent and others being avirulent on applicable hosts (Kema et al. 2000). Although this is not unique, a point of interest with this example is how an avirulent interaction does not necessarily result in death of the avirulent strain, and a plant simultaneously colonized by both avirulent and virulent strains of
Z. tritici can be the site for sexual recombination of the two (Kema et al. 2018; Ware 2006). Kema et al. (2018) further reported that
Z. tritici strains sensitive to stress, including those avirulent on a resistant host and those sensitive to fungicides (e.g., azoxystrobin sensitivity in the presence of its application), participate in sexual reproduction exclusively via paternal parenthood (i.e., spermatia donation only, whereas compatible strains insensitive to the stress provide viable ascogonia). This in turn influences the genetic distribution of ascospores from such crosses, which can both maintain the presence of avirulence genes in a population (decreasing degradation of host resistance) and increase the rate to approximately 100% of passing on maternally transferred alleles from virulent or strobilurin-resistant strains, including
cytb containing the G143A mutation conferring resistance to strobilurins (increasing spread of resistance to applicable fungicides) (Ishii and Hollomon 2015; Kema et al. 2018; Röhr et al. 1999). In cases such as this, less visible avirulent populations can have more readily visible implications for management of the collective pathosystem. Although selection pressure for strobilurin resistance is strong by itself (Torriani et al. 2008) and strobilurin use can at times be common, the rapid and widespread development of strobilurin-resistant
Z. tritici populations in Western Europe and
Pseudocercospora fijiensis (causal agent of black Sigatoka disease on banana) populations in the Caribbean has been attributed to maternal inheritance (Amil et al. 2007; Arango Isaza et al. 2016; Kema et al. 2018; Ware 2006).
Along these lines, resistant ascospores could be produced and spread soon after an initial self-incompatible strain develops resistance, provided a compatible mating type is present, rather than requiring separate developments in two compatible mating types. Further work demonstrating the capacity for analogous sexual reproduction of avirulent or fungicide-sensitive strains with corresponding virulent or resistant strains in additional systems (including
P. fijiensis) could better document how prevalent this phenomenon is and may assist in guiding regional recommendations to potentially prolong compound efficacy in the field (Amil et al. 2007). Although it may currently be unclear if
Z. tritici prioritizes resource distribution in such a manner, stress or reduced fitness has been reported to preferentially allocate more resources in
Aspergillus nidulans to sexual reproduction (Schoustra et al. 2010) and has analogously been suggested to induce a parasexual cycle in
Candida albicans (Berman and Hadany 2012). In regard to different systems involving pathogens such as
Phytophthora infestans or
Botrytis cinerea, the implications of such sexual reproduction (i.e., reproduction of avirulent + virulent or fungicide-sensitive + resistant strains) may arguably play less of a significant role, given the efficiency of asexual reproduction and subsequent capacity for airborne dissemination of asexual sporangia or conidia (more than hundreds of meters) in those systems (Fry 2008; Hahn 2014; Ristaino 2012), as well as movement of infected plant material (e.g., potato tubers for the former system). Nevertheless, for systems in which avirulent or fungicide-sensitive strains may have epidemiological relevance, continued use of sentinel plots and fungicide screening studies (Hsiang et al. 1997; Kelly et al. 2015; Kousik and Ritchie 1999; Sudisha et al. 2005) will be valuable in documenting and providing access to strain diversity data for potentially estimating trait development and dissemination rates under varying management practices.
Spatiotemporal Interactions
Broadly considering plant disease epidemiology, it is the study of a “change in disease intensity in a host population over time and space” (Madden et al. 2007). A vast number of epidemiological studies conducted to date have studied changes at the plant, field, region (and so on) macro scale (Beute and Rodríguez-Kabana 1981; Camann et al. 1995; Carisse et al. 2013; Dalla Lana et al. 2015; Phipps and Beute 1977; Pruvost et al. 2002; Savary and Van Santen 1992), answering such pertinent questions as: how fast does disease develop under varying conditions, or how many plants are at risk and how long do I have before intervention is needed? Interplant studies will continue to be invaluable in mitigating disease loss and informing management decisions.
On the other hand, an area of epidemiology that comparatively has been less investigated to date, and thus has ample room for further exploration, is that of intraplant spatiotemporal dynamics (although one could argue aspects of this to be better considered disease physiology). An example we may consider to illustrate this point is the case of Australian pine and
Sphaeropsis sapinea. Part of a work from Blodgett et al. (2007) has shown that trees inoculated with
S. sapinea at the base exhibited increased (systemic induced) resistance in the upper portion of the stem but also exhibited increased (systemic induced) susceptibility in its shoot tips. The shoot tips becoming more susceptible has implications for the spread of this disease, because spores produced on and spread from these tissues by windblown and splashing rain, pending actual tree size, branch architecture, and location of additional hosts, could have a greater chance of coming into contact with additional hosts (i.e., shoot tips on distal portions of branches are further from the center of a tree and may be closer to another host), although tissue location may be less influential for spores spread by insects or pruning equipment (Swart and Wingfield 1991). Just as with the interplant spread of a pathogen across a field or production region, changes in the susceptibility of the individual spatially explicit parts of a host owing to previous infection (here, not considering ontogenic resistance) could impact the way this disease might spread among groups of trees. Being able to incorporate susceptibility variability in different parts of a host as infections progress increases the spatial and temporal resolution of “hosts” across a given area. Along these lines, we could see the concept of susceptible-exposed-infected-removed models (Chan and Jeger 1994; Jeger et al. 1998; Li and Muldowney 1995) being adapted and applied to individual plant tissues within a population of plants to trace and anticipate disease progression.
As with any additional potentially exploitable information, we must first be able to access it before we can make use of it. Continued surveys, both traditional and more automated or remote sourced, will continue to help increase access to data on what is occurring in the field or given environment. The above example illustrates how one pathogen can influence the spatial susceptibility of one host’s tissues. Similarly, as referenced previously in “The More the Merrier?” section, separate pathogens co-infecting the same plant can likewise influence each other’s rate or extent of spatial progression through the plant. Sometimes these encounters can lead to territorial separations, as is the case with closely related viruses (e.g., as in Takeshita et al. 2004). On the other hand, in a system separate from overcoming the resistance in the previously mentioned Anastasia example, they can also lead to systemic movement of viruses that previously did not cause systemic infections (Choi et al. 2002; Murphy and Kyle 1995; Pieczarka and Zitter 1981). These latter two examples can have important effects on the amount of host tissue available for pathogen access and, consequently, impact the chance a visiting vector might pick up a resident virus or two (Froissart et al. 2010; Mascia et al. 2010). On the prediction side of things, models could be structured to transiently take into account modifying single-system predictions in the presence of applicable multiple-infection epidemics. Where applicable, mapping out an epidemiological-interactome even on the phenotype level of spatiotemporal plant and pathogen responses to individual and multiple pests could provide a further source of information for modeling epidemics under varying conditions. The following section presents one potential means that could have utility in gauging the possible presence of unspecified factors that might include multiple interacting pests.
We Need Information,
STAT(s)!
Statistics and leveraging numerical data are arguably integral to and inseparable from epidemiology, providing insight into biological phenomena that can be quantified. Hence, further developments in statistics and related fields can be anticipated to open additional avenues as to how we might gain understanding of diseases in motion—and, consequently, our ability to manage them—using data and information that we already have access to. Considering an oversimplified history of the progression of statistics and models in plant disease epidemiology, the simplest models contained only fixed effects (e.g., disease severity predicted by temperature and wetness duration) (Beaumont 1947). Later, models were expanded to include factors as random effects (covariance terms in mixed models) (Laird and Ware 1982). These random effects generally serve to adjust the fitted model in one way or another based on different levels of the random effect (e.g., if year is the random effect, the model adjustment in year 1 might be a decrease, whereas in year 2 the adjustment might be an increase). Responses (disease severity, for example) were initially limited to being modeled according to the normal (bell-curve-shaped Gaussian) distribution (Madden and Hughes 1995). Contemporary generalized and mixture modeling methods have in relatively recent history provided the framework for modeling responses according to alternative distributions that allow us to more properly take into account varying mean-variance relationships characteristic of specific types of data (Poisson or negative binomial distributions for count data, for example) (Madden and Hughes 1995; Schabenberger and Pierce 2002).
Taking these previous advancements into account and thinking about room for potential future advancement in plant disease epidemiology begs the question of whether or not this idea of alternative distribution specification might also advantageously be applied toward random effects, which to date in the plant pathological literature have been largely limited to normal distribution specification, which is also the default in common statistics programs (Carisse et al. 2013; Dalla Lana et al. 2015;
R Core Team 2013;
SAS Institute Inc. 2011; Schabenberger and Pierce 2002). As it turns out, specification of alternative random effect distributions has been utilized in allied fields such as statistics and human pathology for several years (Bandyopadhyay et al. 2012; Bock and Aitkin 1981; Zeger and Karim 1991).
Without getting too entrenched with theory, the normal distribution has been the predominant distribution for random effects for several reasons, including computational convenience and biological appropriateness (i.e., levels of random effects, for example, years or replications, represent only a subsample of the greater possible realizations of that factor, and over all values of such a factor we would anticipate it to have no net effect on the response [Schabenberger and Pierce 2002; Zeger and Karim 1991]). Although a search of the literature can produce several examples that make a case for superiority of nonnormal random effect distributions in certain cases (e.g., Ghidey et al. 2004; Ho and Lin 2010; Verbeke and Lesaffre 1996; Zhang and Davidian 2001), for our purposes here, let us assume that for certain or many biological factors, random effects are still optimally described by a bell-shaped curve. If an alternative random effect distribution, on the other hand, happens to provide a better fit of the overall model to the data, it could mean one of two things. First, this may indicate that the normal model specification would be suboptimal and that biologically the random effect is best modeled with an alternative distribution. However, let us continue with the normal random effect as still biologically optimal overall. This leads us to a possible second scenario—one in which there may be further factors at play not specified in the model that are influencing the results. In the case of this second scenario, we can see the utility of nonnormal random effect distributions in providing additional model diagnostics and possibly drawing attention to phenomena behind the data, in turn guiding further hypotheses or potential exploration. These might be directed toward reexamining the data to see if there might be missing factors from the model or if a varied random effect distribution hints at the possible existence of functional subpopulations within the data. These usages fall in line with the example idea of advances in epidemiology serving to increase our interpretation of data that is already available (or accessible).
As a reverse-engineered example of these ideas, we can visit some previously published data regarding in vitro exposure of
X. citri subsp.
citri to combinations of temperature, disinfectant, and treatment duration in a water bath (Anco et al. 2014). Let us imagine we are interested in looking at the mortality responses from the bacteria treated at 50°C. From the originally presented data, it is evident that with increasing treatment duration, the amount of
X. citri subsp.
citri recovered falls on a straight-line response for each disinfectant treatment. Let us now knowingly introduce a wrench into the gears of the analysis. What if during the course of the study the details of whether a disinfectant treatment was included was mistakenly recorded as additional replication numbers? In this case, instead of there being three replications, six experimental replications were noted and details of different disinfectant treatments were excluded. We would still be able to determine a linear response to the data (as shown in
Figure 3), but now the data in this constructed example have more variability, which in and of itself is not unusual. However, with our knowledge of the existence of the excluded disinfectant factor (here, a proxy for a hypothetical interacting pathogen or pest missing from the model), we can see how the combined data and fitted model misses an important feature. If we now take this same intentionally reorganized example dataset with its reclassified replication levels and examine the same model but this time allow the random effect to assume a more flexible distribution (i.e., a semi-nonparametric random effect density as in Vock et al. 2014), we position ourselves to possibly interpret the data from additional angles. As the flexibility of the distribution describing replication increases, it in this case begins to exhibit a bimodal or split geometry. Continuing with the anticipation that replication should overall assume a normal bell-shaped curve as a random effect, a possible interpretation of a potentially better fitting bimodal distribution would be that a factor is missing from the model as specified. This can be a diagnostic clue of sorts suggesting that the data and model may need to be reexamined to determine what might be influencing the random effect’s structure.
In this “reverse-engineered” example, it is easy to go back and see how the omission of the disinfectant term essentially took two distinct groups and lumped them together. More generally speaking, such an approach in evaluating model–data relationships may be valuable in cases in which only a relatively limited portion of an epidemic’s story is gathered through available data, which could in turn guide further hypotheses geared toward examining the presence of missing factors (which could include SSAs) or possible functional subpopulations in the data. As with many things, it helps to have the right tool for the job, and having more statistical tools and information at our disposal can only enhance our potential ability to increase our interpretation and use of already (and not yet) existing data.
|
Figure 3. Data plots for
Xanthomonas citri subsp.
citri alternative
random effect distribution example. In panel
A, black symbols
are estimated bacterial recovery (log[colony forming units + 1])
after in vitro treatment at 50°C, error bars are 95% confidence intervals
of the mean of three replications, and the green line is the fitted regression
curve for the pooled data excluding distinction by presence or absence of
a disinfectant treatment. Panels
B–D display the random effect density
of the replication term allowing for three levels of flexibility (B has the
least flexibility, and
D has the most flexibility). |
Concluding Remarks
As illustrated by the above examples, co-infections from pathogens, or co-involvement of multiple pests, near and far in relation can influence plant disease epidemic progression in meaningful ways. Acknowledgements and concerns over this concept date back throughout the previous century, and continued work to date reflects the sustained relevance to plant disease management in the present going forward. Accordingly, as further knowledge of individual and interacting pathosystems continues to increase, our ability to utilize and coordinate information related to pests perhaps once considered auxiliary can only grow.
References
Amil, A.
F., Heaney, S.
P., Stanger, C., and Shaw, M.
W. 2007. Dynamics of QoI sensitivity in
Mycosphaerella fijiensis in Costa Rica during 2000 to 2003. Phytopathology 97:1451-1457.
Anco, D.
J., Poole, G.
H., and Gottwald, T.
R. 2014. Effects of postharvest treatments on recovery of
Xanthomonas citri subsp.
citri in infected grapefruit leaves. Crop Protection 62:115-123.
Arango Isaza, R. E., Diaz-Trujillo, C., Dhillon, B., Aerts, A., Carlier, J., Crane, C. F., de Jong, T. V., de Vries, I., Dietrich, R., Farmer, A. D., Fereira, C. F., Garcia, S., Guzman, M., Hamelin, R. C., Lindquist, E. A., Mehrabi, R., Quiros, O., Schmutz, J., Shapiro, H., Reynolds, E., Scalliet, G., Souza, M., Stergiopoulos, I., Van der Lee, T. A. J., De Wit, P. J. G. M., Zapater, M.-F., Zwiers, L.-H., Grigoriev, I. V., Goodwin, S. B., and Kema, G. H. J. 2016. Combating a global threat to a clonal crop: Banana black Sigatoka pathogen
Pseudocercospora fijiensis (synonym
Mycosphaerella fijiensis) genomes reveal clues for disease control. PLoS Genet. 12:e1005876.
https://doi.org/10.1371/journal.pgen.1005876
Bandyopadhyay, D., Lachos, V.
H., Castro, L.
M., and Dey, D.
K. 2012. Skew-normal/independent linear mixed models for censored responses with applications to HIV viral loads. Biometrical J. 54:405-425.
Beaumont, A. 1947. The dependence on the weather of the dates of outbreak of potato blight epidemics. Trans. Brit. Mycol. Soc. 31:45-53.
Bergeson, G.
B. 1972. Concepts of nematode-fungus associations in plant disease complexes: A review. Exp. Parasitol. 32:301-314.
Berman, J., and Hadany, L. 2012. Does stress induce (para)sex? Implications for
Candida albicans evolution. Trends Genet. 28:197-203.
Beute, M.
K., and Rodríguez-Kabana, R. 1981. Effect of soil moisture, temperature, and field environment on survival of
Sclerotium rolfsii in Alabama and North Carolina. Phytopathology 71:1293-1296.
Blodgett, J.
T., Eyles, A., and Bonello, P. 2007. Organ-dependent induction of systemic resistance and systemic susceptibility in
Pinus nigra inoculated with
Sphaeropsis sapinea and
Diplodia scrobiculata. Tree Physiol. 27:511-517.
Bock, R.
D., and Aitkin, M. 1981. Marginal maximum-likelihood estimation of item parameters: Application of an EM algorithm. Psychometrika 46:443-459.
Camann, M.
A., Culbreath, A.
K., Pickering, J., Todd, J.
W., and Demski, J.
W. 1995. Spatial and temporal patterns of spotted wilt epidemics in peanut. Phytopathology 85:879-885.
Carisse, O., Morissette-Thomas, V., and Van der Heyden, H. 2013. Lagged association between powdery mildew leaf severity, airborne inoculum, weather, and crop losses in strawberry. Phytopathology 103:811-821.
Chan, M.-S., and Jeger, M.
J. 1994. An analytical model of plant virus disease dynamics with roguing and replanting. J. Appl. Ecol. 31:413-427.
Choi, S.
K., Yoon, J.
Y., Ryu, K.
H., Choi, J.
K., Paukaitis, P., and Park, W.
M. 2002. Systemic movement of a movement-deficient strain of
Cucumber mosaic virus in zucchini squash is facilitated by a cucurbit-infecting potyvirus. J. Gen. Virol. 83:3173-3178.
Dalla Lana, F., Ziegelmann, P.
K., Maia, A.
H.
N., Godoy, C.
V., and Del Ponte, E.
M. 2015. Meta-analysis of the relationship between crop yield and soybean rust severity. Phytopathology 105:307-315.
EPPO. 2005.
Tomato chlorosis crinivirus. EPPO Bull. 35:439-441.
EPPO. 2013.
Tomato chlorosis virus and Tomato infectious chlorosis virus. EPPO Bull. 43:462-470.
Fawcett, H.
S. 1931. The importance of investigations of the effects of known mixtures of microorganisms. Phytopathology 21:545-550.
Froissart, R., Doumayrou, J., Vuillaume, F., Alizon, S., and Michalakis, Y. 2010. The virulence-transmission trade-off in vector-borne plant viruses: A review of (non-)existing studies. Phil. Trans. R. Soc. B 365:1907-1918.
Fry, W. 2008.
Phytophthora infestans: The plant (and
R gene) destroyer. Mol. Plant Pathol. 9:385-402.
García-Cano, E., Resende, R. O., Fernández-Muñoz, R., and Moriones, E. 2006. Synergistic interaction between
Tomato chlorosis virus and
Tomato spotted wilt virus results in breakdown of resistance in tomato. Phytopathology 96:1263-1269.
Ghidey, W., Lesaffre, E., and Eilers, P. 2004. Smooth random effects distribution in a linear mixed model. Biometrics 60:945-953.
Gottwald, T., and Graham, J.
H. 2005. Citrus canker. Plant Health Instructor. Online publication.
https://doi.org/10.1094/PHI-I-2000-1002-01
Hahn, M. 2014. The rising threat of fungicide resistance in plant pathogenic fungi:
Botrytis as a case study. J. Chem. Biol. 7:133-141.
Ho, H., and Lin, T. 2010. Robust linear mixed models using the skew
t distribution with application to schizophrenia data. Biom. J. 52:449-469.
Horsfall, J.
G., and Cowling, E.
B. 1979. Plant Disease: An Advanced Treatise: How Pathogens Induce Disease. Academic Press, New York, NY.
Hsiang, T., Yang, L., and Barton, W. 1997. Baseline sensitivity and cross-resistance to demethylation-inhibiting fungicides in Ontario isolates of
Sclerotinia homoeocarpa. E. J. Plant Pathol. 103:409-416.
Hunsberger, A., Gabel, K., and Mannion, C. 2006. Citrus leafminer (Phyllocnistis citrella). University of Florida IFAS Extension, Gainesville, FL.
Ishii, H., and Hollomon, D.
W. 2015. Fungicide Resistance in Plant Pathogens: Principles and a Guide to Practical Management. Springer, Tokyo, Japan.
Jeger, M.
J., van den Bosch, F., Madden, L.
V., and Holt, J. 1998. A model for analyzing plant-virus transmission characteristics and epidemic development. Math. Med. Biol. 15:1-18.
Jesus Junior, W. C., Belasque Júnior, J., Amorim, L., Christiano, R. S. C., Parra, J. R. P., and Bergamin Filho, A. 2006. Injuries caused by citrus leafminer (Phyllocnistis citrella) exacerbate citrus canker (Xanthomonas axonopodis pv.
citri) infection. Fitopatol. Bras. 31:277-283.
Jesus Junior, W.
C., Paula Junior, T.
J., Lehner, M.
S., and Hau, B. 2014. Interactions between foliar diseases: Concepts and epidemiological approaches. Tropical Plant Pathol. 39:1-18.
Johnson, K.
B. 1990. Assessing multiple pest populations and their effects on crop yield. Pages 203-213 in: Crop Loss Assessments in Rice. IRRI, Los Banos, The Philippines.
Kelly, H.
Y., Dufault, N.
S., Walker, D.
R., Isard, S.
A., Schneider, R.
W., Giesler, L.
J., Wright, D.
L., Marois, J.
J., and Hartman, G.
L. 2015. From select agent to an established pathogen: The response to
Phakopsora pachyrhizi (soybean rust) in North America. Phytopathology 105:905-916.
Kema, G.
H.
J., Gohari, A.
M., Aouini, L., Gibriel, H.
A.
Y., Ware, S.
B., van den Bosch, F., Manning-Smith, R., Alonso-Chavez, V., Helps, J., M’Barek, S.
B., Mehrabi, R., Diaz-Trujillo, C., Zamani, E., Schouten, H.
J., van der Lee, T.
A.
J., Wallwijk, C., de Waard, M.
A., de Wit, P.
J.
G.
M., Verstappen, E.
C.
P., Thomma, B.
P.
H.
J., Meijer, H.
J.
G., and Seidl, M.
F. 2018. Stress and sexual reproduction affect the dynamics of the wheat pathogen effector AvrStb6 and strobilurin resistance. Nature Genet. 50:375-380.
Kema, G.
H.
J., Verstappen, E.
C.
P., and Waalwijk, C. 2000. Avirulence in the wheat Septoria tritici leaf blotch fungus
Mycosphaerella graminicola is controlled by a single locus.
Mol. Plant-Microbe Interact.
13:1375-1379.
Kousik, C.
S., and Ritchie, D.
F. 1999. Development of bacterial spot on near-isogenic lines of bell pepper carrying gene pyramids composed of defeated major resistance genes. Phytopathology 89:1066-1072.
Kranz, J., and Jörg, E. 1989. The synecological approach in plant disease epidemiology. Rev. Tropical Plant Pathol. 6:27-38.
Laird, N.
M., and Ware, J.
H. 1982. Random-effects models for longitudinal data. Biometrics 38:963-974.
Li, M.
Y., and Muldowney, J.
S. 1995. Global stability for the SEIR model in epidemiology. Math. Biosci. 125:155-164.
Madden, L.
V., and Hughes, G. 1995. Plant disease incidence: Distributions, heterogeneity, and temporal analysis. Annu. Rev. Phytopathol. 33:529-564.
Madden, L.
V., Hughes, G., and van den Bosch, F. 2007. The Study of Plant Disease Epidemics. APS Press, St. Paul, MN.
Mascia, T., Cillo, F., Fanelli, V., Finetti-Sialer, M.
M., de Stradis, A., Palukaitis, P., and Gallitelli, D. 2010. Characterization of the interactions between
Cucumber mosaic virus and
Potato virus Y in mixed infections in tomato. Mol. Plant Microbe Interact. 23:1514-1524.
McDonald, M.
C., McDonald, B.
A., and Solomon, P.
S. 2015. Recent advances in the
Zymoseptoria tritici-wheat interaction: Insights from pathogenomics. Front. Plant Sci. 24:1-5.
Murphy, J.
F., and Kyle, M.
M. 1995. Alleviation of restricted systemic spread of pepper mottle potyvirus in
Capsicum annuum cv. Avelar by coinfection with a cucumovirus. Phytopathology 85:561-566.
Orlob, G.
B. 1973. Ancient and medieval plant pathology. Pflanzenschutz-Nachrichten 26:65-294.
Phipps, P.
M., and Beute, M.
K. 1977. Influence of soil temperature and moisture on the severity of Cylindrocladium black rot in peanut. Phytopathology 67:1104-1107.
Pieczarka, D.
J., and Zitter, T.
A. 1981. Effect of interaction between two viruses and
Rhizoctonia on pepper. Plant Dis. 64:404-406.
Ponomarenko, A., Goodwin, S.
B., and Kema, G.
H.
J. 2011. Septoria tritici blotch (STB) of wheat.
Plant Health Instructor. Online publication.
https://doi.org/10.1094/PHI-I-2011-0407-01
Powell, N.
T. 1971. Interactions between nematodes and fungi in disease complexes. Annu. Rev. Phytopathol. 9:253-274.
Pruvost, O., Boher, B., Brocherieux, C., Nicole, M., and Chiroleu, F. 2002. Survival of
Xanthomonas axonopodis pv.
citri in leaf lesions under tropical environmental conditions and simulated splash dispersal of inoculum. Phytopathology 92:336-346.
R Core Team. 2013. R: A language and environment for statistical computing. R Foundation for Statistical Computing, Vienna, Austria.
Ristaino, J.
B. 2012. Worldwide migrations, host shifts, and reemergence of
Phytophthora infestans, the plant destroyer. Pages 192-207 in: Evolution of Virulence in Eukaryotic Microbes. L.
D. Sybley, B.
J. Howlett, and J. Heitman, eds. Wiley, Hoboken, NJ.
Röhr, H., Kües, U., and Stahl, U. 1999. Recombination:Organelle DNA of plants and fungi: Inheritance and recombination. Pages 37-87 in: K. Esser, J. W. Kadereit, U. Lüttge, and M. Runge, eds. Progress in Botany, Vol. 60. Springer, Berlin, Germany.
SAS Institute Inc. 2011. SAS/STAT 9.3 user’s guide. SAS Institute Inc., Cary, NC.
Savary, S., and Van Santen, G. 1992. Effect of crop age on primary gradients of late leaf spot (Cercosporidium personatum) on groundnut. Plant Pathol. 41:265-273.
Savary, S., and Zadoks, J.
C. 1992. Analysis of crop loss in the multiple pathosystem groundnut-rust-leaf-spot. I. Six experiments. Crop Protect. 11:99-109.
Schabenberger, O., and Pierce, F.
J. 2002. Contemporary Statistical Models for the Plant and Soil Sciences. CRC Press, Boca Raton, FL.
Schoustra, S., Rundle, H.
D., Dali, R., and Kassen, R. 2010. Fitness-associated sexual reproduction in a filamentous fungus. Curr. Biol. 20:1350-1355.
Schumann, G.
L., and D’Arcy, C.
J. 2006. Essential Plant Pathology. APS Press, St. Paul, MN.
Sherwood, J.
L., German, T.
L., Moyer, J.
W., and Ullman, D.
E. 2009. Tomato spotted wilt.
Plant Health Instructor. Online publication.
Srinivasan, R., and Alvarez, J.
M. 2007. Effect of mixed viral infections (Potato virus Y-Potato leafroll virus) on biology and preference of vectors
Myzus persicae and
Macrosiphum euphorbiae (Hemiptera: Aphididae). J. Econ. Entomol. 100:646-655.
Styller, J. 2012. Facilitative and antagonistic interactions between plant viruses in mixed infections. Mol. Plant Pathol. 13:204-216.
Styller, J., and Grupa, A. 2016. Antagonistic within-host interactions between plant viruses: Molecular basis and impact on viral and host fitness. Mol. Plant Pathol. 17:769-782.
Sudisha, J., Amruthesh, K.
N., Deepak, S.
A., Shetty, N.
P., Sarosh, B.
R., and Shetty, H.
S. 2005. Comparative efficacy of strobilurin fungicides against downy mildew disease of pearl millet. Pestic. Biochem. Physiol. 81:188-197.
Swart, W.
J., and Wingfield, M.
J. 1991. Biology and control of
Sphaeropsis sapinea on
Pinus species in South Africa. Plant Dis. 75:761-766.
Takeshita, M., Shigemune, N., Kikuhara, K., Furuya, N., and Takanami, Y. 2004. Spatial analysis for exclusive interactions between subgroups I and II of
Cucumber mosaic virus in cowpea. Virology 328:45-51.
Tatineni, S., Graybosch, R. A., Hein, G. L., Wegulo, S. N., and French, R. 2010. Wheat cultivar-specific disease synergism and alteration of virus accumulation during co-infection with
Wheat streak mosaic virus and
Triticum mosaic virus. Phytopathology 100:230-238.
Thrall, P. H., Barrett, L. G., Dodds, P. N., and Burdon, J. J. 2016. Epidemiological and evolutionary outcomes in gene-for-gene and matching allele models. Front. Plant Sci. 6:1084.
Torriani, S. F. F., Brunner, P. C., McDonald, B. A., and Sierotzki, H. 2008. QoI resistance emerged independently at least 4 times in European populations of
Mycosphaerella graminicola. Pest Manag. Sci. 65:155-162.
Verbeke, G., and Lesaffre, E. 1996. A linear mixed-effects model with heterogeneity in the random-effects population. J. Am. Stat. Assoc. 91:217-221.
Vock, D.
M., Davidian, M., and Tsiatis, A.
A. 2014. SNP_NLMM: A SAS macro to implement a flexible random effects density for generalized linear and nonlinear mixed models. J. Stat. Software 56:1-21.
Wallace, H.
R. 1978. The diagnosis of plant diseases of complex etiology. Ann. Rev. Phytopathol. 16:379-402.
Ware, S.
B. 2006. Aspects of Sexual Reproduction in
Mycosphaerella Species on Wheat and Barley: Genetic Studies on Specificity, Mapping, and Fungicide Resistance. Ph.D. thesis, Wageningen University, The Netherlands.
Wintermantel, W. M., Cortez, A. A., Anchieta, A. G., Gulati-Sakhuja, A., and Hladky, L. L. 2008. Co-infection by two criniviruses alters accumulation of each virus in a host-specific manner and influences efficiency of virus transmission. Phytopathology 98:1340-1345.
Zeger, S.
L., and Karim, M.
R. 1991. Generalized linear models with random effects: A Gibbs sampling approach. J. Am. Stat. Assoc. 86:79-86.
Zeigler, R.
S., Leong, S.
A., and Teng, P.
S. 1994. Rice Blast Disease. CAB International, Wallingford, Oxfordshire, U.K.
Zhang, D., and Davidian, M. 2001. Linear mixed models with flexible distributions of random effects for longitudinal data. Biometrics 57:795-802.