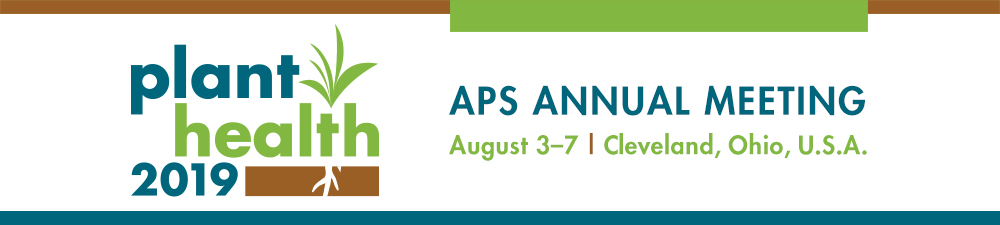
TECHNICAL SESSION: Predictive Disease Modeling
Big data solutions for smallholder farmers in Southeast Asia: machine learning for real-time forecasting of cassava mosaic disease risk
Kelsey Andersen - Plant Pathology Department, University of Florida. Nami Minato- Niigata University, Erik Delaquis- International Center for Tropical Agriculture (CIAT), James Legg- International Institute of Tropical Agriculture, Karen Garrett- Plant Pathology Department, University of Florida, Cu Thi Le Thuy- Internatio
Cassava mosaic viruses, cause of cassava mosaic disease (CMD), are a major threat to global cassava yields. Sri Lankan cassava mosaic virus (SLCMV) was first reported in Cambodia in 2015 and more recently in Vietnam and China, and with symptoms reported in Thailand. Risk of dispersal of this pathogen is twofold; it is vectored locally by whiteflies and also through the movement of infected cassava planting material, in the form of stakes. Stakes are transported long distances with regular movement across national boundaries. We present a computational framework for integrating disease incidence, climate, weather, land use, and social data layers using machine learning methods to predict and map regional CMD establishment risk. Model outcomes were used in scenario analyses with multilayer network models to predict the locations which should be targeted for surveillance and clean seed deployment. Data for parameterizing the stake trade network include household surveys conducted in Cambodia and Vietnam. Interestingly, locations that were optimal for surveillance were not necessarily optimal for the deployment of clean seed material, a practical finding for epidemic mitigation in the region. Additionally, we discuss risk pathways that may be important for the introduction of future disease threats for the region. The methods presented here provide a framework for modeling emerging pathogens and pests across systems.